Empower Your Business with RAG Generative Ai Expertise
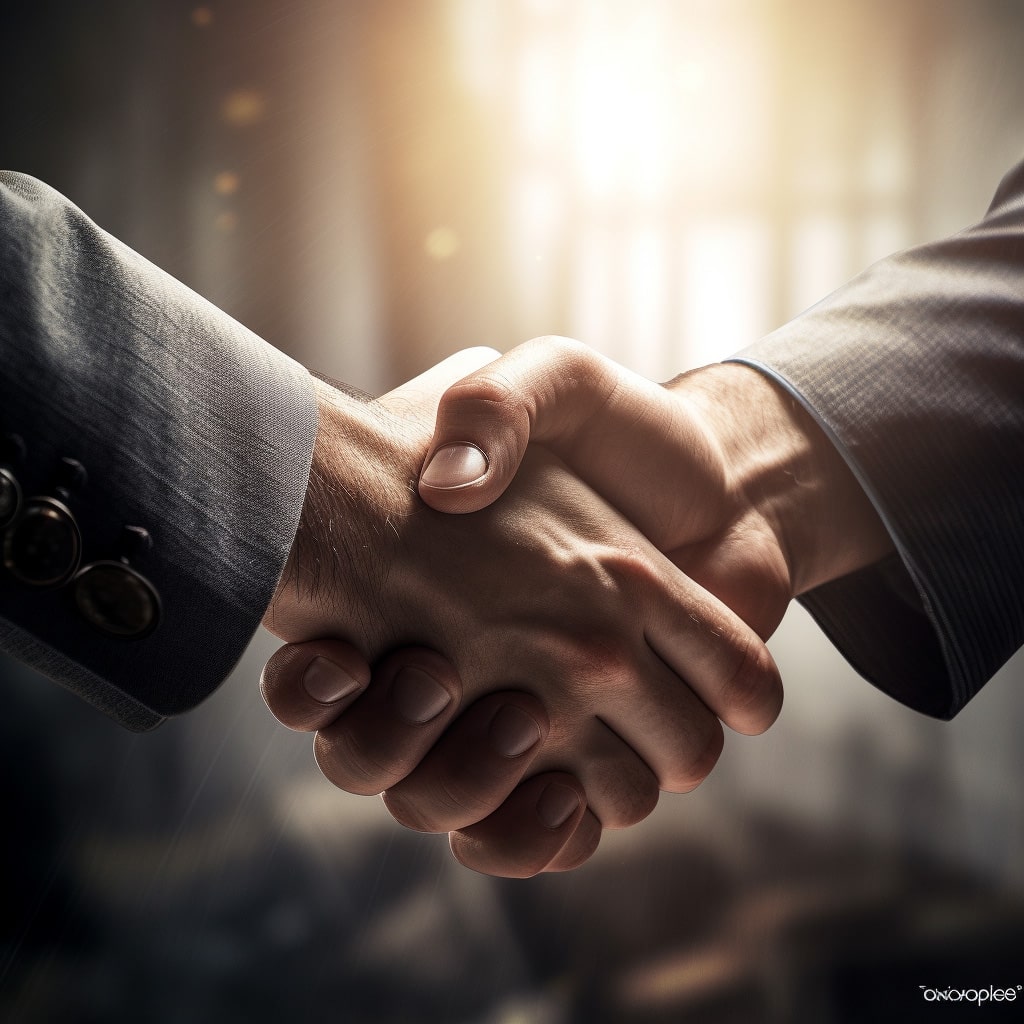
What is retrieval augmented generation (RAG)?
Retrieval Augmented Generation (RAG) is a cutting-edge approach that combines the power of retrieval-based language models with generative AI techniques. At EnhancedAi, we define RAG as the synergy between retrieving relevant information and generating contextually accurate responses. This approach allows businesses to tap into the vast knowledge stored in language models while maintaining precision and relevance in their AI-generated content.
What are the benefits of using RAG?
Improved accuracy
Reduced bias
Increased creativity
What are the use cases for RAG?
Content generation
Machine translation
Question answering
Code generation
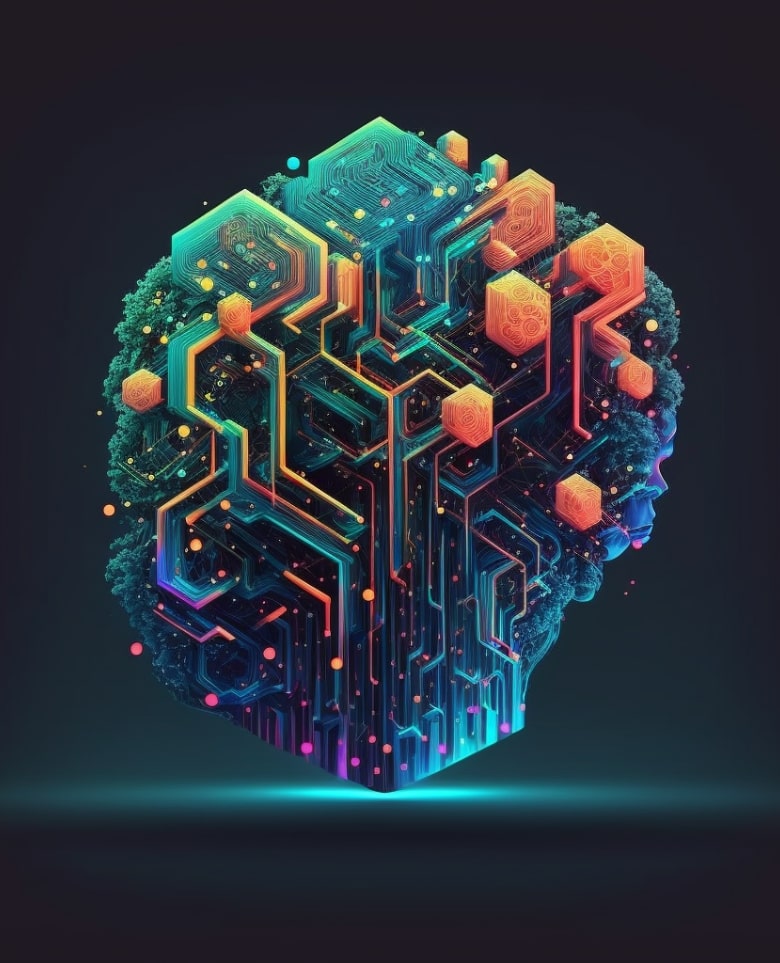
What are the challenges of using RAG?
While RAG offers immense potential, it also presents several challenges:
- Data quality: RAG models heavily depend on the quality of the data they retrieve. Ensuring access to reliable and up-to-date information sources is crucial.
- Integration complexity: Implementing RAG into existing systems can be complex. Businesses often face challenges when integrating RAG into their workflows and applications.
- Training and customization: Fine-tuning RAG models for specific business needs requires expertise. Companies may struggle to optimize models for their unique use cases.
- Ethical considerations: Like all AI systems, RAG models must be used responsibly to avoid perpetuating biases or generating inappropriate content.
What are the different types of RAG models?
RAG has a broad range of applications in business, including content creation, customer support, data analysis, and more. EnhancedAi specializes in tailoring RAG solutions to suit your specific business needs, offering consulting services that guide you through the integration process.
Active RAG models
Passive RAG models
What are the key features of a good RAG model?
01. Accuracy
A reliable RAG model must provide accurate and contextually relevant information in its responses.
02. Fluency
The generated content should be fluent and coherent, ensuring a seamless user experience.
03. Creativity
RAG models should strike a balance between accuracy and creativity, allowing for innovative content generation.
04. Efficiency
Efficient RAG models can generate content quickly and at scale, making them valuable assets for businesses with high content demands.
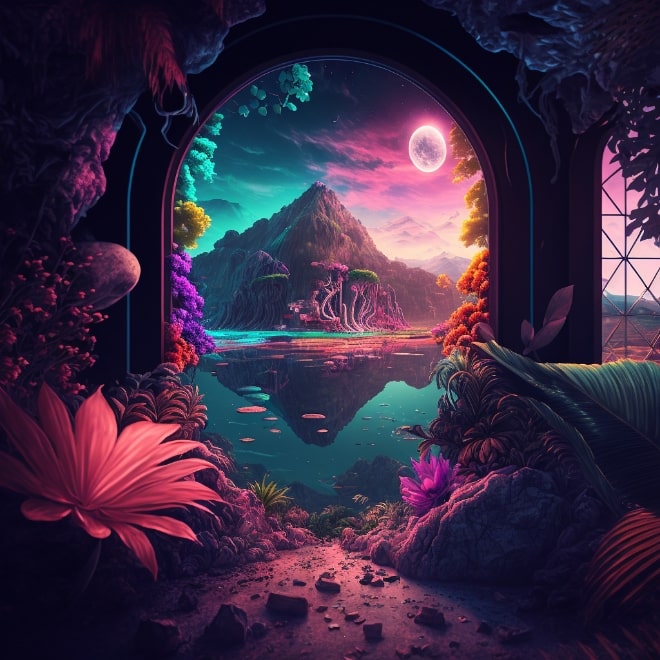
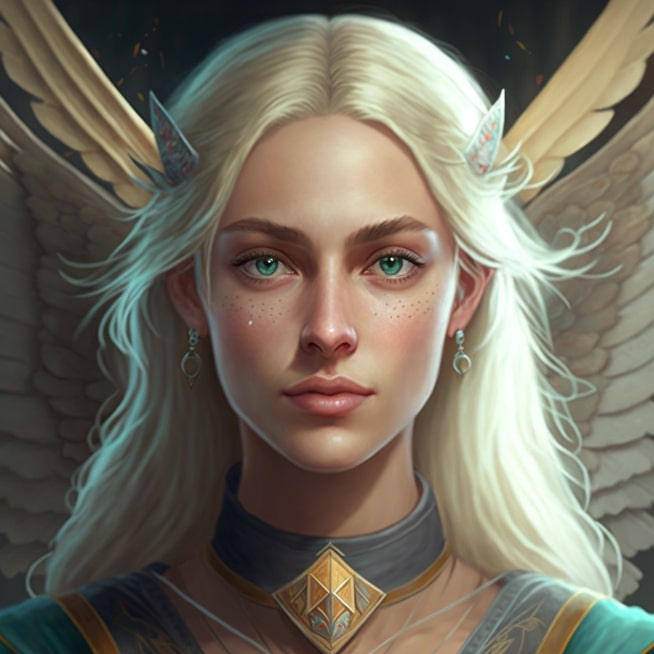
At EnhancedAi, our mission is to empower businesses with the transformative potential of RAG. We understand the challenges you face in adopting and implementing this cutting-edge technology, and we are here to guide you every step of the way. Here’s how EnhancedAi can help you unlock the full benefits of RAG:
Expert Consulting
Customization
Integration Services
Ongoing Support
Responsible AI
Training and Education
Scalability
With EnhancedAi as your partner, you can confidently embrace RAG technology and lead the way in harnessing its potential. Contact us today to discover how RAG can transform your business, and let us guide you on the journey to AI-powered success.
FAQ
What is retrieval augmented generation (RAG)?
Retrieval augmented generation (RAG) is a technique that combines retrieval and generation models to improve the performance of natural language processing (NLP) tasks. RAG models work by first retrieving relevant information from a large knowledge base, and then using that information to generate a response. This approach can lead to more accurate and informative responses than traditional generation models, which are limited by their own knowledge and understanding.
What are the benefits of using RAG?
RAG offers a number of benefits over traditional generation models, including:
- Improved accuracy and informativeness: RAG models can generate more accurate and informative responses by leveraging the knowledge and information contained in a large knowledge base.
- Reduced hallucinations: RAG models are less likely to generate hallucinations, which are false or misleading responses.
- Increased domain specificity: RAG models can be customized to specific domains by using domain-specific knowledge bases.
What are the use cases for RAG?
RAG can be used for a variety of NLP tasks, including:
- Question answering: RAG can be used to generate answers to questions by retrieving relevant information from a knowledge base and then summarizing it in a clear and concise way.
- Document summarization: RAG can be used to generate summaries of documents by extracting key information and then presenting it in a condensed form.
- Chatbots: RAG can be used to power chatbots that can provide informative and engaging conversations with users.
What are the challenges of using RAG?
RAG models can be computationally expensive to train and deploy. Additionally, they require access to a large and high-quality knowledge base.
What is the future of RAG?
RAG is a relatively new technology, but it has the potential to revolutionize the field of NLP. RAG models are becoming increasingly powerful and accessible, and they are being used for a wide range of applications. As RAG technology continues to develop, we can expect to see it used in even more innovative and impactful ways.
How can RAG be used in business?
RAG can be used in business in a number of ways, including:
- Customer service: RAG can be used to power chatbots that can provide customer support and answer customer questions.
- Marketing: RAG can be used to generate personalized marketing campaigns and product recommendations.
- Sales: RAG can be used to generate leads and qualify prospects.
- Product development: RAG can be used to generate product ideas and feedback from customers.
- Research and development: RAG can be used to generate new hypotheses and insights.
What are the different types of RAG models?
There are two main types of RAG models:
- Retrieval-based language models: These models retrieve information from a knowledge base and then use that information to generate a response.
- Generative AI: These models generate text from scratch, but they can be improved by using information from a knowledge base.
What are the key features of a good RAG model?
A good RAG model should have the following features:
- Accuracy: The model should be able to generate accurate and informative responses.
- Fluency: The model’s responses should be fluent and easy to read.
- Comprehensiveness: The model should be able to generate responses that are comprehensive and cover all aspects of the query.
- Relevance: The model’s responses should be relevant to the query.
- Diversity: The model should be able to generate a variety of different responses to the same query.
What are some of the best-known RAG models?
Some of the best-known RAG models include:
- BART: BART is a retrieval-based language model that was developed by Google AI.
- T5: T5 is a generative AI model that was developed by Google AI.
- RAG-Transformer: RAG-Transformer is a RAG model that was developed by Google AI.